In a crucial advancement for the state of the art of robotics computing, Acceleration Robotics has contributed the RobotPerf™ benchmark suite, a new standard for assessing robotics computing performance. Current alpha release benchmarks selected solutions from key leading players including AMD, Intel, Qualcomm, and NVIDIA, laying the foundation for improved, efficient, and effective robotics systems, thereby pushing the boundaries of what's possible in robotics today.
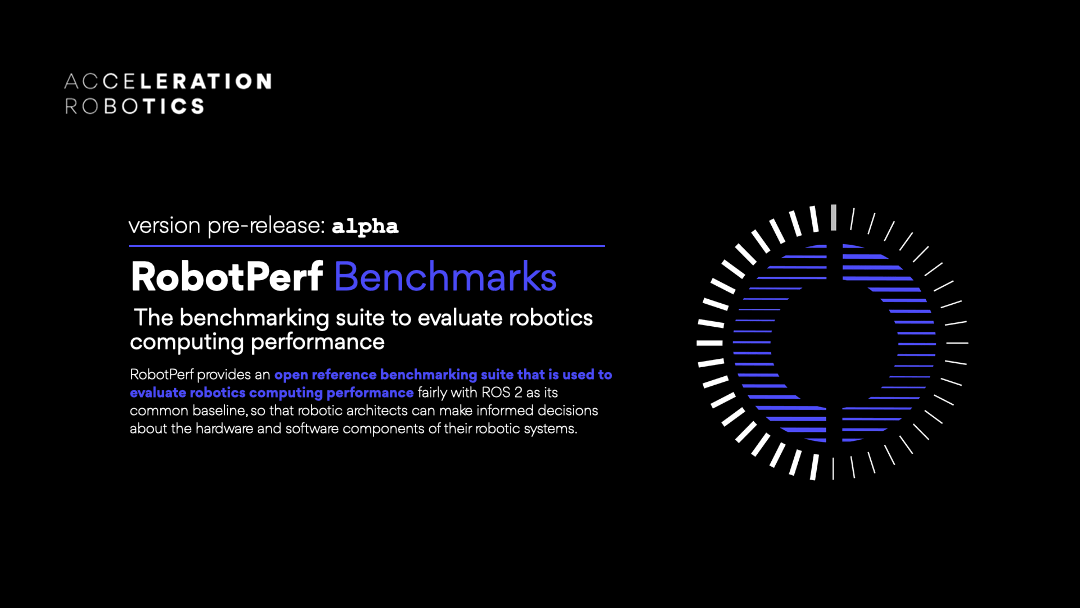
A few months after introducing the project, we are absolutely thrilled to announce our significant contribution to the RobotPerf™ benchmark suite. The outcome is unmistakable — Acceleration Robotics has elevated the standard in robotics computing performance by contributing and enhancing this open, fair, and technology-agnostic benchmarking platform.
Being spearheaded by Acceleration Robotics and Harvard University's Edge Computing Lab, RobotPerf™ aims to become a consortium of robotics leaders from industry, academia, and research labs, committed to creating unbiased benchmarks that enable robotic architects to compare the performance of various robotics computing components.
In a field as multifaceted and dynamic as robotics, the RobotPerf™ benchmark suite has emerged as the gold standard for assessing robotics performance, pushing the boundaries of what's possible in robotics today
Our contribution is setting a new precedent in the robotics industry.
Enhancing Robotics Performance, through Performance Benchmarks for Robots
Our notable contribution to the RobotPerf benchmarking suite highlights the continuing evolution in robotics computing performance. The benchmarking suite, designed to test the robustness of various hardware components such as CPU, GPU, FPGA, and other compute accelerators, brings out the potential versatility and adaptability of robotics systems across diverse robotic computing workloads including perception, localization, control, manipulation, navigation, and more to come.
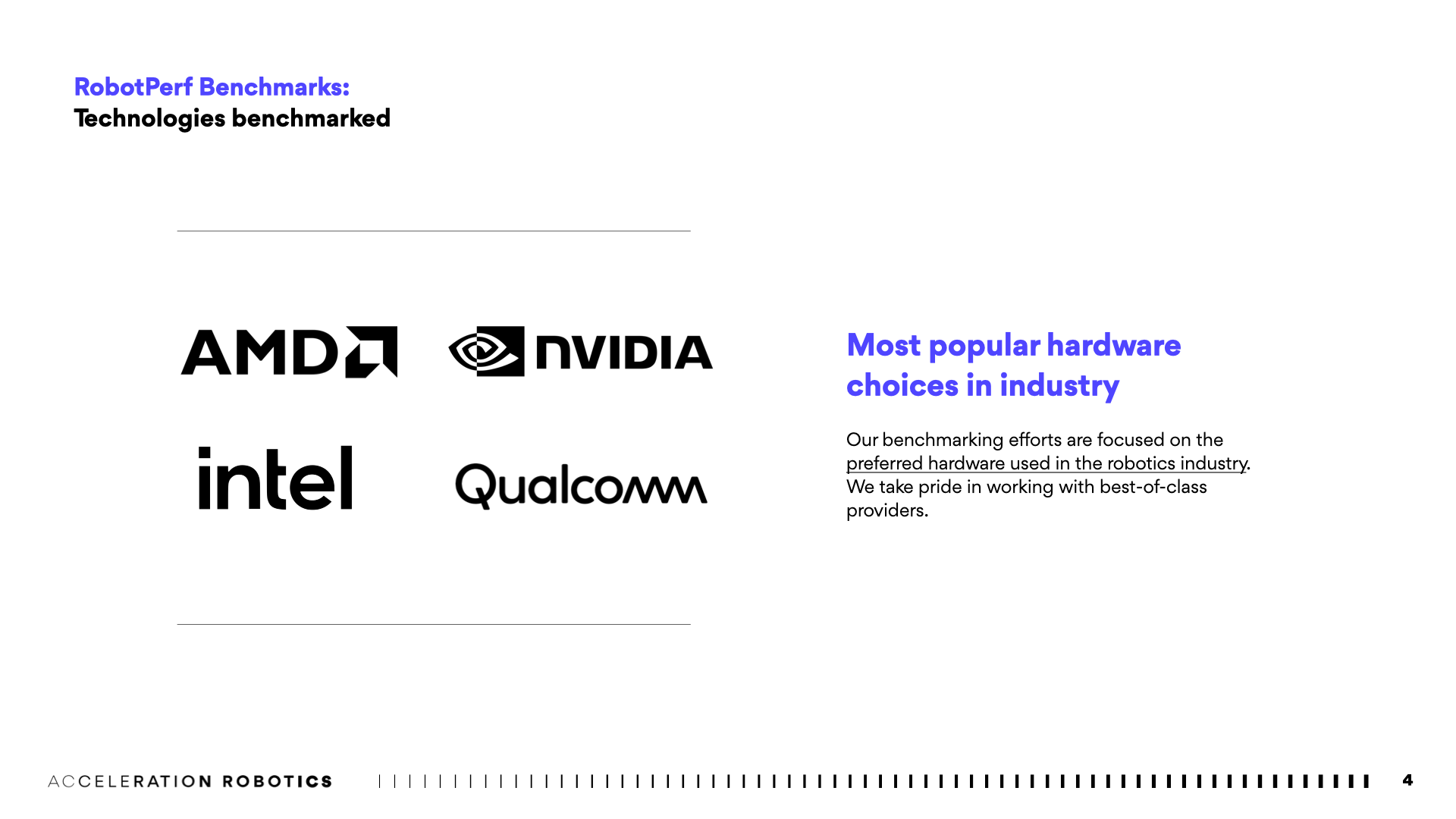
This suite, importantly, is evaluated with the technology from top silicon vendors in robotics, including AMD, Intel, Qualcomm, and NVIDIA. Our improvements in this arena underpin the robustness of our technology and our engineering team's commitment to meet the challenge head-on and deliver a truly representative and reproducible performance benchmarking suite.
The Implications of the RobotPerf™ Benchmarking Process
The RobotPerf™ benchmarks operate on ROS 2, the widely accepted standard for robot application development. The suite provides reproducible and representative performance evaluations of a robotic system's computing capabilities.
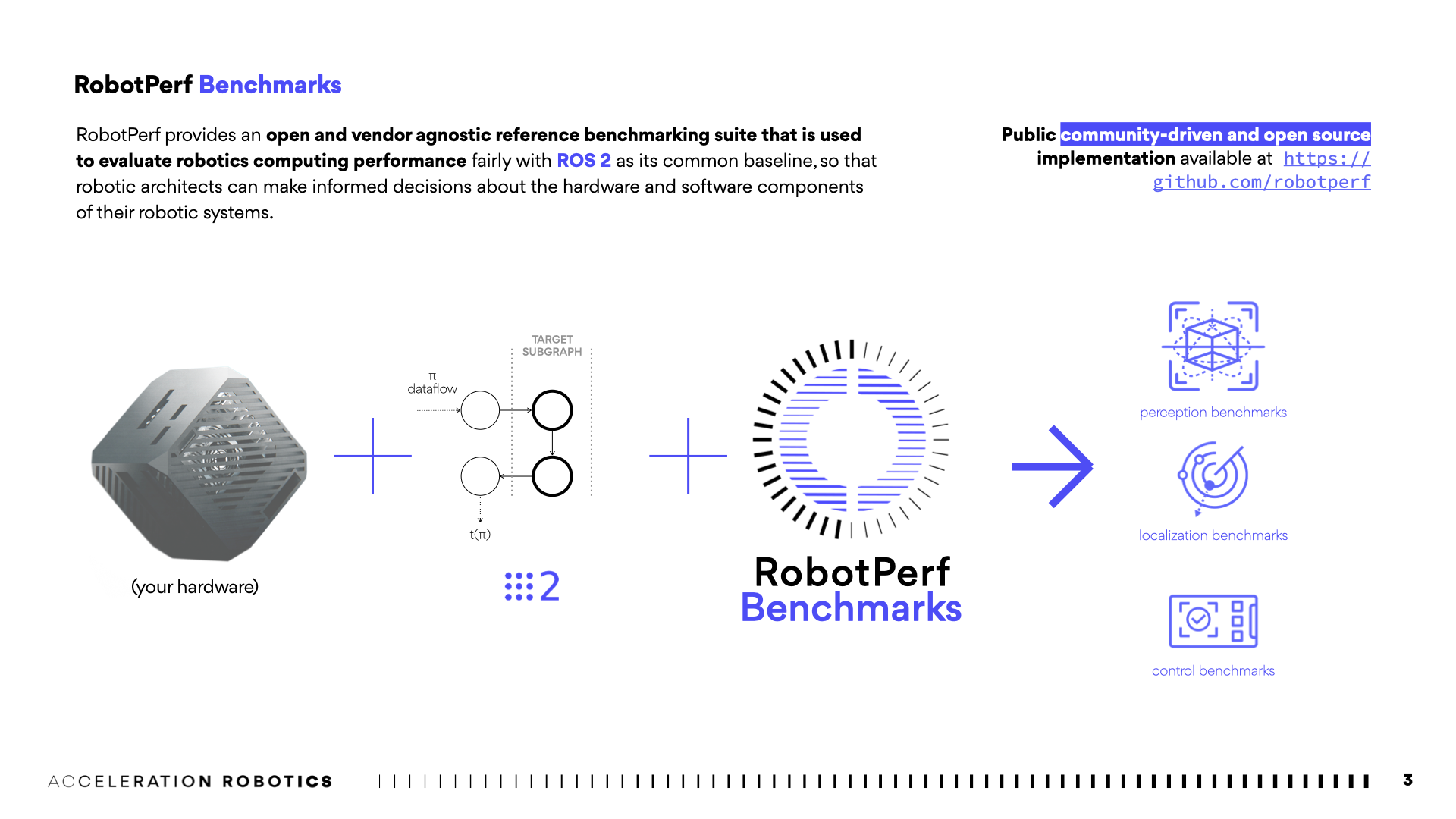
RobotPerf™ benchmark will be conducting periodic releases, each of which will include latest accepted results. For the RobotPerf™ result evaluation, each and any participant can provide input to the project using the RobotPerf benchmarks GitHub repository. Once all participants complete their individual testing, the results will be peer-reviewed by a committee group for accuracy and industry-guide.
Setting a New Industry Standard in Robotics Benchmarking
So, what does our contribution to the RobotPerf™ benchmark suite signify?
In essence, we have made strides towards facilitating a more comprehensive assessment of the performance of robotics computing components. This is vital in an industry where performance directly impacts the practical efficiency and efficacy of robotics in real-world applications. Our contribution will allow industry players to better compare and evaluate the performance of their technologies, in turn leading to advancements in the field. In particular, we've enabled performance benchmarks in robotic systems through two benchmarking approaches: grey-box
and black-box
benchmarks.
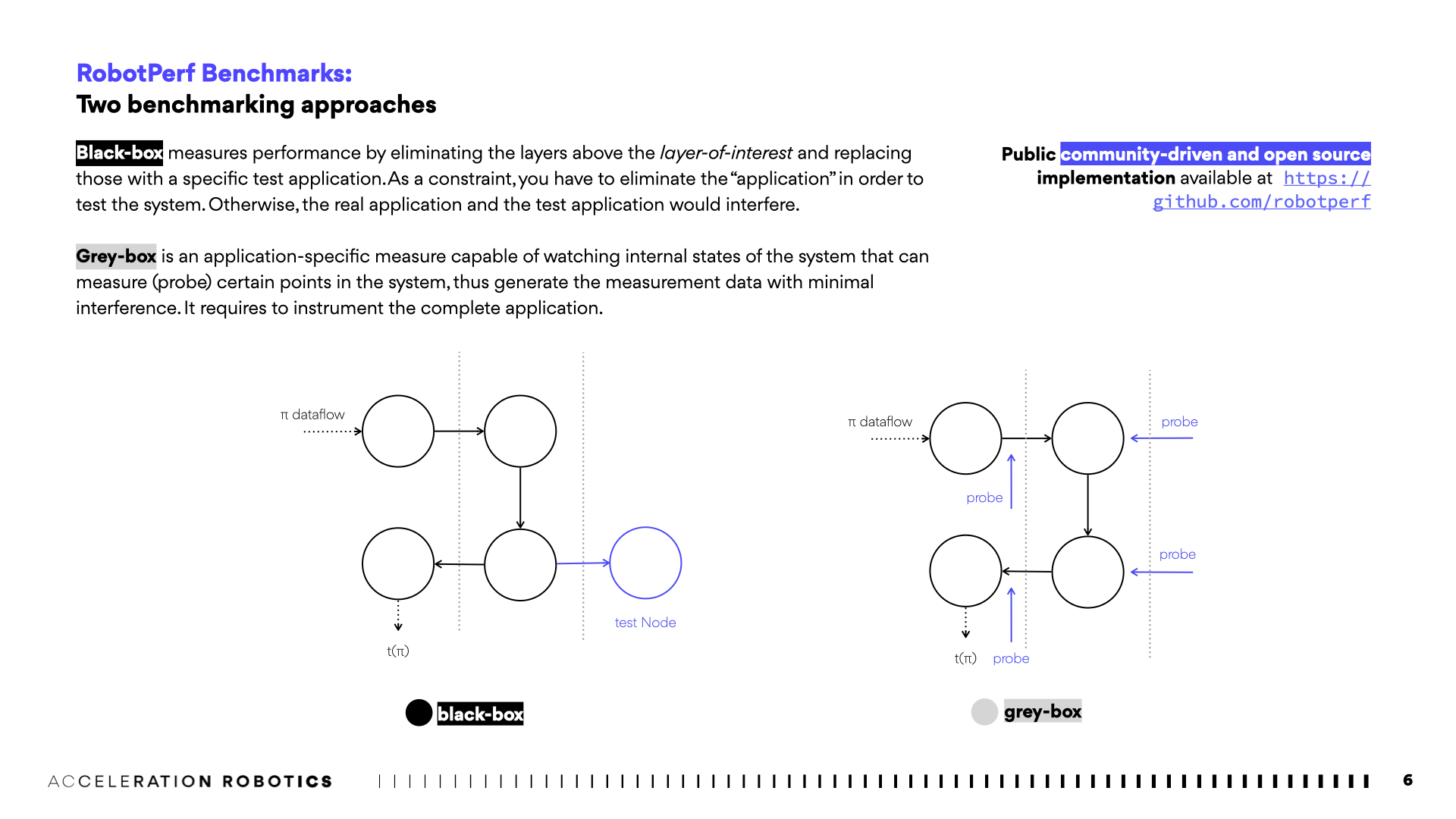
Black-Box Benchmarking: A Layer-focused Approach
Black-box benchmarking provides a unique approach to performance evaluation by eliminating the layers above the layer-of-interest, replacing these with a specific test application. This layer-focused method gives a distinct view of the performance metrics of the system.
However, the constraints of this approach lie in the necessity to eliminate the "application" for testing the system. Without this, the original application and the test application could interfere, leading to potentially skewed results. This type of benchmarking effectively assesses the performance of individual components within a system without the potential complexities introduced by the broader system.
Grey-Box Benchmarking: Harnessing Internal Insights
On the other hand, Grey-box benchmarking takes a more application-specific approach. It’s capable of observing the internal states of the system, measuring specific points in the system, and thereby generating performance data with minimal interference. This involves instrumenting the entire application, a detailed process that provides a comprehensive view of system performance.
Grey-box benchmarking is powerful for its ability to provide insights that might not be apparent in a purely external evaluation. By instrumenting the complete application, Grey-box benchmarking can yield data that directly ties to the performance of the system in real-world scenarios, resulting in a more thorough and nuanced performance evaluation.
In conclusion, both Black-box and Grey-box benchmarks play pivotal roles in RobotPerf™, providing different perspectives on robotics computing performance. These benchmarks, when used effectively, can provide a comprehensive and insightful view of system performance, leading to improvements and enhancements in the field of robotics.
The Significance for the Industry and Our Partners
With the diverse combinations of robotics hardware and software, there's a pressing need for an accurate and reliable standard of performance. And Acceleration Robotics has stepped up to the plate. Our enhancements to the benchmarking suite provide a tangible way for roboticists and technology vendors to evaluate their systems, understand trade-offs between different algorithms, and ultimately build more effective robotics systems. Preliminary results collected across benchmarks will have the following format:
RobotPerf alpha : a1 latency |
RobotPerf alpha : a1 power |
RobotPerf alpha : a1 throughput |
---|---|---|
![]() |
![]() |
![]() |
All data and results are available in the RobotPerf's Github benchmark
repository.
The advancements we've made in the RobotPerf™ benchmarking suite represent a pivotal moment for our partners and the robotics industry. Our work is set to drive forward improvements in hardware acceleration for robotics and robotics computing performance, making it easier for developers and engineers to create more efficient, effective, and adaptable robotics computing systems.
In making these contributions, we're pushing the boundaries of what's possible in robotics. We're thrilled about the potential impact this could have on the industry and we look forward to continuing our work with the RobotPerf™ consortium. If you're interested to participate and collaborate, join us!